Biotech is leaving AI opportunity on the table. Data and culture are the fix
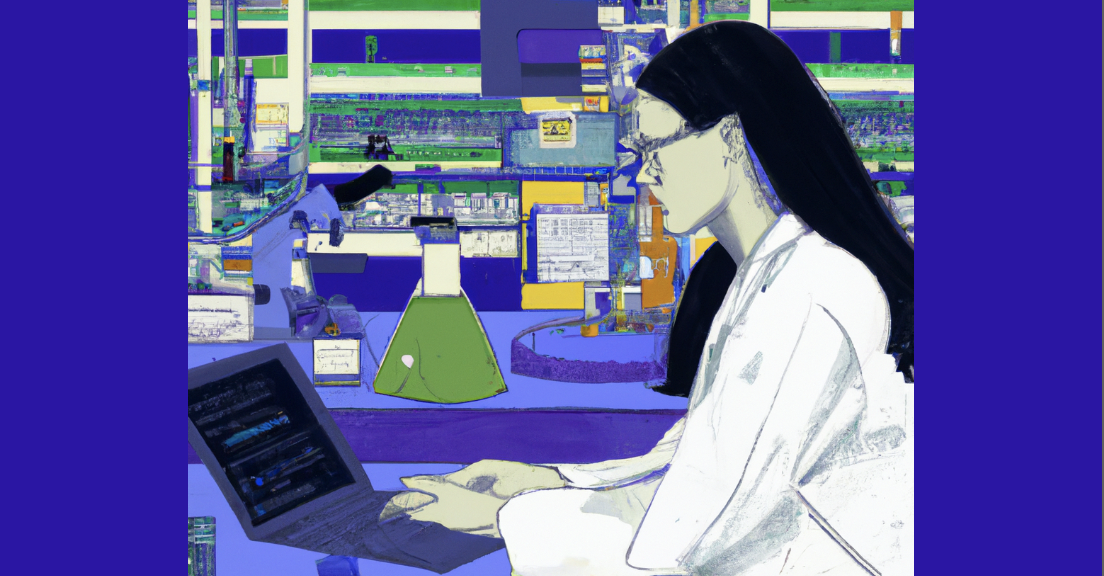
In January 2020, Exscientia announced the first artificial intelligence-designed drug to enter Phase 1 clinical trials. The drug, a treatment for obsessive compulsive disorder, took 12 months to reach Phase 1, compared to the industry norm of over five years. During Covid, machine learning proved itself: AbCellera sorted through 5M cells in one week to identify an antibody to be mass produced. With Moderna, AI did not design the Covid vaccine, but optimized it. Using its tech platform, Moderna learned from producing 20,000 mRNA sequences, which helped it design and manufacture the first batch of its Covid vaccine for testing in just 42 days.
Across industries, AI and ML technologies are advancing rapidly and having profound impacts. Biology, with its inherently rich and complex datasets, is no exception.
However, progress and success with AI and ML in biotech is not the norm. Adoption across the industry has been slow and difficult to operationalize. What is leading to breakthroughs for early leaders, while leaving others treading water?
The common factors underlying ML success are a solid data foundation and a company culture that supports and values FAIR data (findable, accessible, interoperable, reusable). The promise that AI and ML presents — to make every stage of R&D exceedingly more efficient — is the forcing function that will drive our industry to invest in data assets and engineering culture. How do we accelerate this outcome, and what is the status quo today?
Swimming in data, struggling with structure
If data is the cornerstone to AI and ML, biotech should seemingly be awash in opportunity. The size of datasets is increasing rapidly with the rise of lab automation technologies, the fast pace at which drug discovery unfolds, and the sheer size of data in fields that do screening and sequencing in high-throughput. It’s predicted that biotech data collection will surpass all other fields, hurtling past even astronomy, to have the largest data volume by 2025.
However, the abundance of data in pharma and the life sciences is widely understood to be a blessing and a curse. This is due to the heterogeneous and hierarchical nature of scientific data, which is perpetuated by how the data is generated, organized, and interpreted.
In part, this pitfall occurs because data modeling is simply trickier in biology. In other fields where ML has momentum, such as online commerce, advertising, finance, or media, data models are easier to pin down and agree upon, industry-wide. This lowers the barrier to storing, standardizing, and analyzing across datasets. Meanwhile, scientific data modeling often varies wildly – even across a single team in an organization. For instance, the featurization of a genome can be debated endlessly, as the optimal representation has complex dependencies on the organism, the biological tools for manipulating them, and how uncertainty and updates are handled.
In addition, training ML models in biology usually requires that you have captured a lot of process data and metadata to fuel models. Scientific observation of how a molecule performs is usually a complex function of how the molecule was produced, isolated, tested. For example, pooling data to measure yield or performance of different proteins likely would have terrible generalizability if you neglect any of tens of potential factors like the organism that produced it, or the scale at which the protein was produced. Models that unknowingly mix apples and oranges typically do not generalize to new data well in biotech.
The mistake that we commonly see is that companies jump ahead, and do data science before solving the hard foundational problems of establishing the pipeline and flow of data for analysis. They don’t pause to operationalize the flow of data coming from experimental pipelines for usage by data scientists. As un-sexy as it is, companies need to build their data strategy and systems before benefitting from ML. Beyond simply collecting data, doing so in a standardized way and anticipating how the data should be consumed, is key. Companies need to design their data systems for the analytics and AI and ML they aim to layer on top.
Data culture is strong in science. Data engineering culture is needed too.
The second challenge is related: Biotech is a field that has struggled to attract experienced talent to manage, structure, and make sense of their data.
To be a data scientist in biotech, you need to be bilingual — understanding the data, engineering, and the hard science, which can span many domains (biology, chemistry, manufacturing, pharmacology, toxicology). Why? Because to create and populate effective data models, you need to understand the complex data types being produced, and anticipate the insights scientists want to extract from them.
Anyone on the leading edge of applying ML and AI for biology can tell you that finding these ‘bilingual unicorns’ is rare. Yet it is essential to find and develop them because data science can only thrive in a company that has an established data engineering culture — it’s a chicken or the egg problem. Without that culture in place, many biotechs find it difficult, if not impossible, to attract and retain the talent needed to set up the data culture that is the foundation of ML and AI. It can even be hard to know what to look for in prospective candidates if you aren’t a unicorn yourself. Similarly, it can be hard to persuade your leadership colleagues to offer fair market compensation for a unicorn if you can find one.
Understandably, the majority of biotech companies do not natively have an engineering culture. Instead, they’re grounded in science. To what extent can we blend the best parts of tech culture and scientific culture to maximize impact?
Consider what makes for a strong engineering or data engineering culture. Partially, it’s systems and tools. At a tech company, engineers are accustomed to having the basic software infrastructure already in place: think code review, continuous integration, cloud compute resources, skilled collaborators to grow with. Engineers are not an isolated function but are instead embedded into the business value. They expect to be supported by leaders who connect the dots between software innovation and company-wide KPIs, thus unlocking value for the team and instilling pride in their work. For the engineers and data scientists who are ‘outsiders’ in the biotech sphere, this is especially critical.
One way biotech companies can set the stage for ML success is in hiring tech leadership from day one. Even five years ago, it was uncommon to hire for tech, data scientists, or data engineer roles in your first 50 hires. Now, it’s common that these skills are part of senior leadership or early hires, especially in the tech-bio and syn-bio movements. I think of Insitro, Relay Therapeutics, and Recursion, to name a few. Data scientists are so critical in biotech that they should be an early hire and play a leadership role, with the opportunity to shape a company’s culture and business decisions from the onset.
Biotechs can also support the systems and tools that accommodate data scientists and engineers. Individuals want to know they’ll be able to make an impact, be set up for success, and enjoy the working environment. This means having the right tools to ingest, house, and analyze scientific data. Talent gravitates towards businesses with good foundations in place, as it’s a signal their work will be valued.
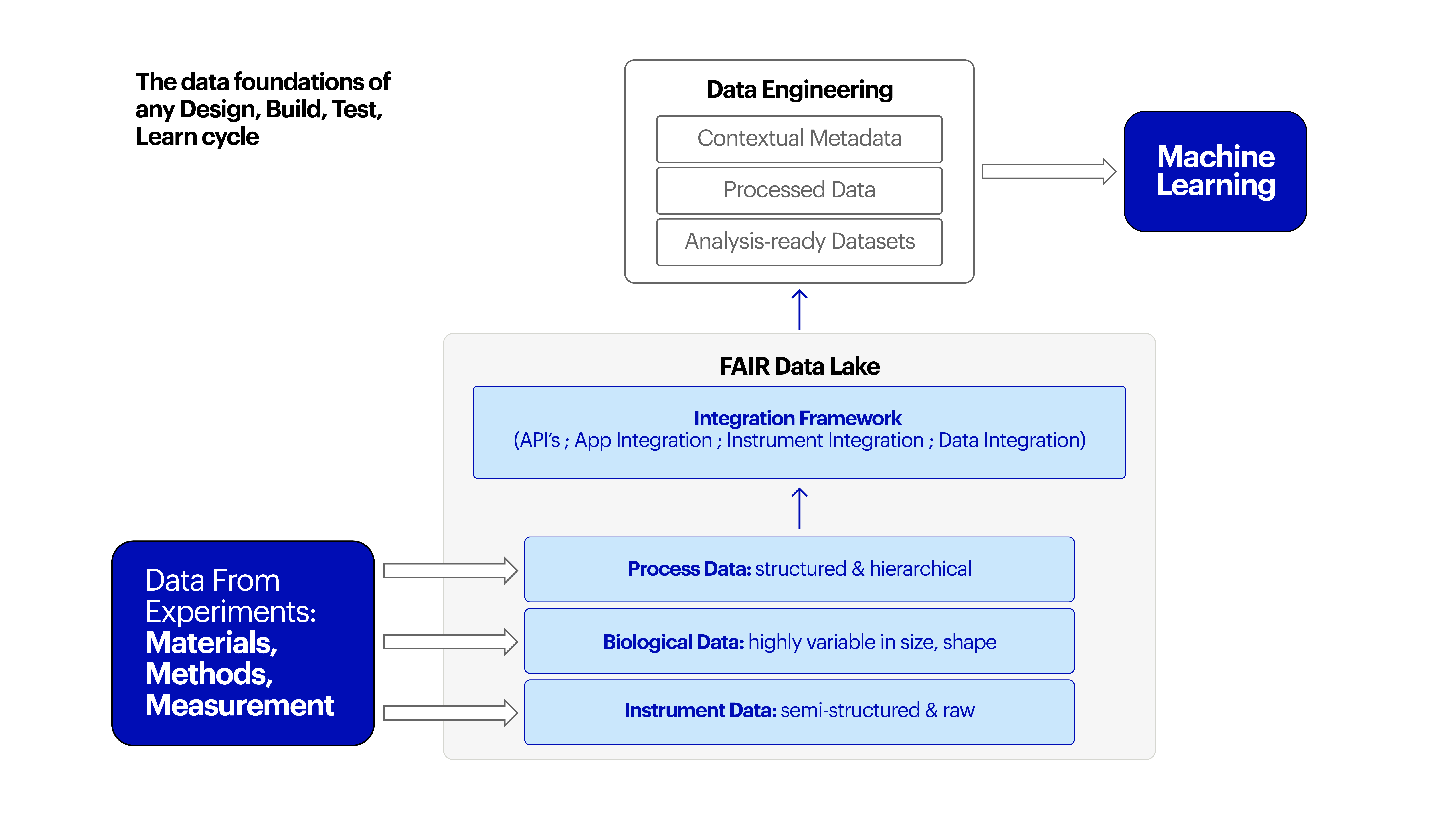
Evolving systems, structure, and culture is challenging, but critical
From research, to discovery, to evidence gathering, and regulatory approval processes, the work of going from molecule to product or drug is manually intensive, repetitive, and prone to human error. ML and AI are not the silver bullet, but instead tools to be used at every step of the R&D process to increase efficiency, reduce distraction, and radically accelerate timelines.
No doubt, it will take longer to realize the full potential of success with AI and ML in biotech. Science by its nature is a more patient process. But the right tools, talent, and culture are the missing ingredients to catalyze progress today.
Janet Matsen, PhD: Janet specializes in building platform machine learning capabilities for biotech.
Helen Liu-Mayo: Helen specializes in Machine Learning Product Development. At Benchling, she’s working on democratizing ML (and improving data standards!) in biotech.
Powering breakthroughs for over 1,300 biotechnology companies, from startups to Fortune 500s